Below is a list of Workshops and Tutorials that should be available to remote attendees in Zoom. It is up to the organizer of the event to initiate streaming in Zoom, so it is possible that not all of these will have active Zoom meetings. Live stream of all plenary events (opening, awards, award candidates, keynotes, panels) takes place on the virtual home page. Live 8.30- 10am and 2-4pm Vancouver time.
[ East 3 ]
[ West 217 - 219 ]
Workshop on Fair, Data Efficient and Trusted Computer Vision will address four critical issues in enhancing user trust in AI and computer vision systems namely: (i) Fairness, (ii) Data Efficient learning and critical aspects of trust including (ii) explainability, (iii) mitigating adversarial attacks robustly and (iv) improve privacy and security in model building with right level of credit assignment to the data sources along with transparency in lineage.
[ East 1 ]
The CVPR MCV workshop provides a unique forum for researchers and developers in academia, industry and healthcare to present, discuss and learn about cutting-edge advances in machine learning and computer vision for medical image analysis and computer assisted interventions. The workshop offers a venue for potential new collaborative efforts, encouraging more dataset and information exchanges for important clinical applications.
The ultimate goal of the MCV workshop is to bring together stakeholders interested in leveraging medical imaging data, machine learning and computer vision algorithms to build the next generation of tools and products to advance image-based healthcare. It is time to deliver!
The program features invited talks from leading researchers from academia and industry and clinicians. There will be no paper submissions at this year's workshop.
[ West 207 ]
The goal of this workshop is to foster research on the next generation of visual perception systems that reason over label spaces that go beyond a list of simple category names. Modern applications of computer vision require systems that understand a full spectrum of labels, from plain category names (“person” or “cat” ), over modifying descriptions using attributes, actions, functions or relations (“women with yellow handbag” , “parked cars” , or “edible item” ), to specific referring descriptions (“the man in the white hat walking next to the fire hydrant” ). Natural language is a promising direction not only to enable such complex label spaces, but also to train such models from multiple datasets with different, and potentially conflicting, label spaces. Besides an excellent list of invited speakers from both academia and industry, the workshop will present the results of the OmniLabel challenge, which we held with our newly collected benchmark dataset that subsumes generic object detection, open-vocabulary detection, and referring expression comprehension into one unified and challenging task.
[ West 103 - 104 ]
Computer vision technologies like generative image models are rapidly being integrated into creative domains to, for example, aid in artistic content retrieval and curation, generate synthetic media, or enable new forms of artistic methods and creations. However, creative AI technologies bring with them a host of ethical concerns, ranging from representational harms associated culturally sensitive matter to impact on artistic practices and copyright and ownership concerns. In particular, it is unclear what kinds of performance failures and biases these models bring when deployed in cross-cultural and non-western settings.
We encourage retrospective discussions, position papers examining the cross-cultural and social impacts of creative applications of computer vision, ethical considerations in this domain including but not limited to artwork attributions, inequity in cultural performance, cultural appropriation, environmental impacts of generative arts, biases embedded in generative arts, dynamics of art marketplaces/platforms, and policy perspectives on creative AI.
Our aim is to create a platform for interdisciplinary discussions on these issues among computer vision researchers, socio-technical researchers, policy makers, social scientists, artists, and other cultural stakeholders. This year our Generative Art Demo will invite artists to use computer vision technologies to create art pieces that center questions and topics of cultural significance and create …
[ East 17 ]
Content moderation (CM) is a rapidly growing need in today’s industry, with a high societal impact, where automated CM systems can discover discrimination, violent acts, hate/toxicity, and much more, on a variety of signals (visual, text/OCR, speech, audio, language, generated content, etc.). Leaving or providing unsafe content on social platforms and devices can cause a variety of harmful consequences, including brand damage to institutions and public figures, erosion of trust in science and government, marginalization of minorities, geo-political conflicts, suicidal thoughts and more. Besides user-generated content, content generated by powerful AI models such as DALL-E and GPT present additional challenges to CM systems.
With the prevalence of multimedia social networking and online gaming, the problem of sensitive content detection and moderation is by nature multimodal. The Hateful memes dataset [1] highlights the multimodal nature of content moderation, for example, an image of a skunk and a sentence “you smell good” are benign/neutral separately, but can be hateful when interpreted together. Another aspect is the complementary nature of multimodal analysis where there may be ambiguity in interpreting individual modalities separately. Moreover, content moderation is contextual and culturally multifaceted, for example, different cultures have different conventions about gestures. This requires CM approach …
[ West 116 ]
The purpose of this workshop is to challenge the computer vision community to develop robust image captioning models that advance the state-of-the-art both in terms of accuracy and fairness (i.e. mitigating societal biases). Both of these issues must be addressed fully before image captioning technology can be reliably deployed in a large-scale setting.
The workshop will focus on testing the true limits of image captioning models under the zero-shot image captioning setting. It aims to challenge the models by providing a large-scale evaluation dataset that includes a larger variety of visual concepts from many domains (including new concepts such as COVID-19) as well as various image types (photographs, illustrations, graphics). To accomplish this task, the models need to broadly understand language-vision relations and also learn how to combine language components for a new concept of image. Before the workshop, a challenge on zero-shot image captioning will be processed, and the results will be shared in the workshop. By providing results only on the limited evaluation dataset, the submitted models will be challenged to understand new concepts and unseen environments.
Throughout the workshop and challenge, we will cover a broad range of topics on understanding language and image together, so that …
[ West 115 ]
The 5th International Workshop on Gaze Estimation and Prediction in the Wild (GAZE 2023) at CVPR 2023 aims to encourage and highlight novel strategies for eye gaze estimation and prediction with a focus on robustness and accuracy in extended parameter spaces, both spatially and temporally. This is expected to be achieved by applying novel neural network architectures, incorporating anatomical insights and constraints, introducing new and challenging datasets, and exploiting multi-modal training. Specifically, the workshop topics include (but are not limited to):
- Reformulating eye detection, gaze estimation, and gaze prediction pipelines with deep networks.
- Applying geometric and anatomical constraints into the training of (sparse or dense) deep networks.
- Leveraging additional cues such as contexts from face region and head pose information.
- Developing adversarial methods to deal with conditions where current methods fail (illumination, appearance, etc.).
- Exploring attention mechanisms to predict the point of regard.
- Designing new accurate measures to account for rapid eye gaze movement.
- Novel methods for temporal gaze estimation and prediction including Bayesian methods.
- Integrating differentiable components into 3D gaze estimation frameworks.
- Robust estimation from different data modalities such as RGB, depth, head pose, and eye region landmarks.
- Generic …
[ East 14 ]
While machine learning (ML) models have achieved great success in many perception applications, concerns have risen about their potential security, robustness, privacy, and transparency issues when applied to real-world applications. Irresponsibly applying a foundation model to mission-critical and human-centric domains can lead to serious misuse, inequity issues, negative economic and environmental impacts, and/or legal and ethical concerns. For example, ML models are often regarded as “black boxes” and can produce unreliable, unpredictable, and unexplainable outcomes, especially under domain shifts or maliciously crafted attacks, challenging the reliability of safety-critical applications; Stable Diffusion may generate NSFW content and privacy violated-content.
This goals of this tutorial are to:
Provide a holistic and complementary overview of trustworthiness issues, including security, robustness, privacy, and societal issues to allow a fresh perspective and some reflection on the induced impacts and responsibility as well as introduce the potential solutions.
Promote awareness of the misuse and potential risks in existing AI techniques and, more importantly, to motivate rethinking of trustworthiness in research.
Present case studies from computer vision-based applications.
This tutorial will provide sufficient background for participants to understand the motivation, research progress, known issues, and ongoing challenges in trustworthy perception systems, in addition to pointers to open-source …
[ East 6 ]
Precise geo-location of a ground image within a large-scale environment is crucial to many applications, including autonomous vehicles, robotics, wide area augmented reality and image search. Localizing the ground image by matching to an aerial/ overhead geo-referenced database has gained noticeable momentum in recent years, due to significant growth in the availability of public aerial/ overhead data with multiple modalities (such as aerial images from Google/ Bing maps, and USGS 2D and 3D data, Aerial LiDAR data, Satellite 3D Data etc.). Matching a ground image to aerial/ overhead data, whose acquisition is simpler and faster, also opens more opportunities to industrial and consumer applications. However, cross-view and cross-modal visual geo-localization comes with additional technical challenges due to dramatic changes in appearance between the ground image and aerial/ overhead database, which capture the same scene differently in time, viewpoints or/and sensor modalities. This tutorial will provide a comprehensive review on the research problem of visual geo-localization, including same-view/cross-time, cross-view, cross-modal settings to both new and experienced researchers. It also provides connection opportunities for the researchers of visual geo-localization and other related fields.
[ East 18 ]
The tutorial will present a comprehensive review of recent advances in (deep) anomaly detection on image and video data. Three major AD paradigms will be discussed, including unsupervised/self-supervised approaches (anomaly-free training data), semi-supervised approaches (few-shot training anomaly examples are available), and weakly-supervised approaches (videl-level labels are available for frame-level detection). Additionally, we will also touch on anomaly segementation tasks, focusing on autonomous driving settings. The tutorial will be ended with a panel discussion on AD challenges and opportunities.
[ East 5 ]
This tutorial will teach attendees how to overcome performance, cost, privacy and robustness challenges when using distributed and federated software systems for learning and deploying Computer Vision and ML applications across various hardware settings (networked machines, GPUs, embedded, mobile systems). The audience will learn about theory, implementation and practice of these topics: state-of-the-art approaches and system architectures, forms of distributed parallelism, pitfalls in the measurement of parallel application performance, parallel ML compilers, computation-communication-memory efficiency in federated learning (FL), trustworthy FL, tackling device heterogeneity in FL, and on-device FL systems.
[ West 212 ]
This tutorial will introduce effective methodologies for re-designing algorithms for efficient content understanding, image generation, and neural rendering. Most importantly, we show how the algorithms can be efficiently deployed on mobile devices, eventually achieving real-time interaction between users and mobile devices.
[ West 208 - 209 ]
Monocular depth estimation (MDE) is an important low-level vision task, with application in fields such as augmented reality, robotics and autonomous vehicles. Recently, there has been an increased interest in self-supervised systems capable of predicting the 3D scene structure without requiring ground-truth LiDAR training data. Automotive data has accelerated the development of these systems, thanks to the vast quantities of data, the ubiquity of stereo camera rigs and the mostly-static world. However, the evaluation process has also remained focused on only the automotive domain and has been largely unchanged since its inception, relying on simple metrics and sparse LiDAR data.
This workshop seeks to answer the following questions:
1. How well do networks generalize beyond their training distribution relative to humans?
2. What metrics provide the most insight into the model’s performance? What is the relative weight of simple cues, e.g. height in the image, in networks and humans?
3. How do the predictions made by the models differ from how humans perceive depth? Are the failure modes the same?
The workshop will therefore consist of two parts: invited keynote talks discussing current developments in MDE and a challenge organized around a novel benchmarking procedure using the SYNS dataset.
[ East 2 ]
Incorporating new knowledge in existing models to adapt to novel problems is a fundamental challenge of computer vision. Humans and animals continuously assimilate new experiences to survive in new environments and to improve in situations already encountered in the past. Moreover, while current computer vision models have to be trained with independent and identically distributed random variables, biological systems incrementally learn from non-stationary data distributions. This ability to learn from continuous streams of data, without interfering with previously acquired knowledge and exhibiting positive transfer is called Continual Learning. The CVPR Workshop on “Continual Learning in Computer Vision” (CLVision) aims to gather researchers and engineers from academia and industry to discuss the latest advances in Continual Learning. In this workshop, there are regular paper presentations, invited speakers, and a technical benchmark challenges to present the current state of the art, as well as the limitations and future directions for Continual Learning, arguably one of the most challenging milestones of AI.
[ East 16 ]
[ West 111 - 112 ]
[ East 12 ]
This tutorial will introduce two open platforms which can significantly accelerate the research in computer vision ——OpenMMLab and OpenDataLab.
OpenMMLab is an open-source algorithm platform for computer vision. It aims to provide a solid benchmark and promote reproducibility for academic research. We have released more than 30 high-quality projects and toolboxes in various research areas such as image classification, object detection, semantic segmentation, action recognition, etc. OpenMMLab has made public more than 300 algorithms and 2,400 checkpoints. Over the past years, OpenMMLab has gained popularity in both academia and industry. It receives over 78,000 stars on GitHub and involves more than 1,700 contributors in the community.
OpenDataLab, which was initially released in March, 2022, is an open data platform for artificial intelligence, especially including a large number of datasets for computer vision.
[ West 211 ]
The attention mechanism has revolutionized deep learning research across many disciplines starting from NLP and expanding to vision, speech, and more. Different from other mechanisms, the elegant and general attention mechanism is easily adaptable and eliminates modality-specific inductive biases. As attention becomes increasingly popular, it is crucial to develop tools to allow researchers to understand and explain the inner workings of the mechanism to facilitate better and more responsible use of it. This tutorial focuses on understanding and interpreting attention in the vision and the multi-modal setting. We present state-of-the-art research on representation probing, interpretability, and attention-based semantic guidance, alongside hands-on demos to facilitate interactivity. Additionally, we discuss open questions arising from recent works and future research directions.
[ West 202 - 204 ]
Diffusion models have been widely adopted in various computer vision applications and are becoming a dominating class of generative models. In the year 2022 alone, diffusion models have been applied to many large-scale text-to-image foundation models, such as DALL-E 2, Imagen, Stable Diffusion and eDiff-I. These developments have also driven novel computer vision applications, such as solving inverse problems, semantic image editing, few-shot textual inversion, prompt-to-prompt editing, and lifting 2d models for 3d generation. This popularity is also reflected in the diffusion models tutorial in CVPR 2022, which has accumulated nearly 60,000 views on YouTube over 8 months. The primary goal of the CVPR 2023 tutorial on diffusion models is to make diffusion models more accessible to a wider computer vision audience and introduce recent developments in diffusion models. We will present successful practices on training and sampling from diffusion models and discuss novel applications that are enabled by diffusion models in the computer vision domain. These discussions will also heavily lean on recent research developments that are released in 2022 and 2023. We hope that this year’s tutorial on diffusion models will attract more computer vision practitioners interested in this topic to make further progress in this exciting area.
[ East 7 ]
[ West 220 - 222 ]
[ East 19 - 20 ]
[ East 11 ]
[ West 110 ]
End-to-end autonomous driving, as a relatively new paradigm (compared to the modular design) yet with great potential, has already attracted attention from both academia and industry. This workshop serves a brand-new perspective to discuss broad areas of end-to-end framework design for autonomous driving on a system-level consideration. Central to the program is a series of invited talks and four new challenges in the self-driving domain. Each challenge combines new perspectives of multiple components in perception and planning compared to conventional pipelines.
[ East Ballroom C ]
The CVPR 2023 Workshop on Autonomous Driving (WAD) aims to gather researchers and engineers from academia and industry to discuss the latest advances in perception for autonomous driving. In this full-day workshop, we will host speakers as well as technical benchmark challenges to present the current state of the art, limitations and future directions in the field - arguably one of the most promising applications of computer vision and artificial intelligence. The previous chapters of this workshop attracted hundreds of researchers to attend. This year, multiple industry sponsors are also joining our organizing efforts to push it to a new level.
[ East 9 ]
Recent years have seen the stunning powers of Visual Language Pre-training (VLP) models. Although VLPs have revolutionalized some fundamental principles of visual language reasoning (VLR), the other remaining problems prevent them from “thinking” like a human being: how to reason the world from breaking into parts (compositionality), how to achieve the generalization towards novel concepts provided a glimpse of demonstrations in context (prompts), and how to debias visual language reasoning by imagining what would have happened in the counterfactual scenarios (causality).
The workshop provides the opportunity to gather researchers from different fields to review the technology trends of the three lines, to better endow VLPs with these reasoning abilities. Our workshop also consists of two multi-modal reasoning challenges under the backgrounds of cross-modal math-word calculation and proving problems. The challenges are practical and highly involved with our issues, therefore, shedding more insights into the new frontiers of visual language reasoning.
[ West 223 - 224 ]
The exploitation of the power of big data in the last few years led to a big step forward in many applications of Computer Vision. However, most of the tasks tackled so far are involving visual modality only, mainly due to the unbalanced number of labelled samples available among modalities (e.g., there are many huge labelled datasets for images while not as many for audio or IMU based classification), resulting in a huge gap in performance when algorithms are trained separately.
Recently, a few works have started to exploit the synchronization of multimodal streams (e.g., audio/video, RGB/depth, RGB/Lidar, visual/text, text/audio) to transfer semantic information from one modality to another reaching surprising results. Interesting applications are also proposed in a self-supervised fashion, where multiple modalities are learning correspondences without need of manual labelling, resulting in a more powerful set of features compared to those learned processing the two modalities separately. Other works have also shown that particular training paradigms allow neural networks to perform well when one of the modalities is missing due to sensor failure or unfavorable environmental conditions. These topics are gaining lots of interest in computer vision community in the recent years.
The information fusion from multiple sensors …
[ East 4 ]
Many biological organisms are evolved to exhibit diverse quintessential behaviors via physical and social interactions with surroundings, and understanding these behaviors is a fundamental goal of multiple disciplines including neuroscience, biology, medicine, behavior science, and sociology. For example, ethogramming characterizes the behavioral states and their transitions, which further provides a scientific basis to understand innate human behaviors, e.g., decision-making, attention, and group behaviors. These analyses require objective, repeatable, and scalable measurements of animal behaviors that are not possible with existing methodologies that leverage manual encoding from animal experts and specialists. Recently, computer vision has been making a groundbreaking impact by providing a new tool that enables computational measurements of the behaviors.
The workshop offers invited talks, orals, and poster sessions by the leading scientists in the field, coming computer vision, neuro science, and biology. Our webpage list the full schedule, accepted papers, and posters.
[ Virtual ]
This tutorial focuses on the challenges of reconstructing a 3D model of a human face followed by generating facial expressions. It comprises three parts, covering facial reconstruction from skeletal remains, 4D dynamic facial performance, and audio-driven talking face generation. Firstly, Face modeling is a fundamental technique and has broad applications in animation, vision, games, and VR. Facial geometries are fundamentally governed by their underlying skull and tissue structures. This session covers a forensic task of facial reconstruction from skeletal remains, in which we will discuss how to restore fragmented skulls, model anthropological features, and reconstruct human faces upon skulls. Then, we will detail how to capture 4D facial performance, which is the foundation for face modeling and rendering. We will consider the hardware designs for cameras, sensors, lighting, and the steps to obtain dynamic facial geometry along with physically-based textures (pore-level diffuse albedo, specular intensity, and normal, etc.,). We will discuss the two complementary workhorses: multi-view stereo and photometric stereo, and the combination with neural rendering advances and medical imaging. Finally, talking face generation will be discussed including 3D animation parameters and 2D photo-realistic video, as well as their applications. It aims to create a talking video of a speaker …
[ East Exhibit Hall A ]
[ East 13 ]
[ East 12 ]
With the recent advances in AR/VR recently a wider range of applications such as virtual touring, Building Information Modeling (BIM), e.g. floorplan generation and 3D holistic understanding have been emerging. Such applications have attracted a lot of interest from both academia and industry and motivated a lot of investments in the form of dataset collection, research, publications and products. A few recent examples of such datasets are Zillow Indoor Dataset (ZInD), Apple’s ARKit Scenes dataset and Facebook’s Habitat-Matterport dataset. The size and unique type of annotations provided by each of these datasets provide a huge opportunity for CV/ML researchers to focus on different aspects of scene and environment understanding beyond what was possible before.
Motivated by the recent release of datasets such as Zillow Indoor Dataset (ZInD), Apple's ARKit Scenes dataset and Facebook's Habitat-Matterport dataset, in this workshop we would like to bring industry and academia together and encourage both to focus on specific under explored aspects of environment understanding. We encourage researchers to go beyond "scene understanding" and explore "environment understanding" with a focus on understanding structure through tasks such as 2D/3D room layout estimation, understanding relation of "rooms" for floorplan generation, localization of media within rooms and floorplans, …
[ West 207 ]
Vision-based detection and recognition studies have been recently achieving highly accurate performance and were able to bridge the gap between research and real-world applications. Beyond these well-explored detection and recognition capabilities of modern algorithms, vision-based forecasting will likely be one of the next big research topics in the field of computer vision. Vision-based prediction is one of the critical capabilities of humans, and the potential success of automatic vision-based forecasting will empower and unlock human-like capabilities in machines and robots.
One important application is in autonomous driving technologies, where a vision-based understanding of a traffic scene and prediction of the movement of traffic actors is a critical piece of the autonomous puzzle. Various sensors such as cameras and lidar are used as the "eyes" of a vehicle, and advanced vision-based algorithms are required to allow safe and effective driving. Another area where vision-based prediction is used is the medical domain, allowing deep understanding and prediction of future medical conditions of patients. However, despite its potential and relevance for real-world applications, visual forecasting or precognition has not been the focus of new theoretical studies and practical applications as much as detection and recognition problems.
Through the organization of this workshop, we …
[ West 111 - 112 ]
The goal of this workshop is to gather researchers, students, and advocates who work at the intersection of accessibility, computer vision, and autonomous and intelligent systems. In particular, we plan to use the workshop to identify challenges and pursue solutions for the current lack of shared and principled development tools for vision-based accessibility systems. For instance, there is a general lack of vision-based benchmarks and methods relevant to accessibility (e.g., people using mobility aids are currently mostly absent from largescale datasets in pedestrian detection). Towards building a community of accessibility-oriented research in computer vision conferences, we also introduce a large-scale fine-grained computer
vision challenge. The challenge involves visual recognition tasks relevant to individuals with disabilities. We aim to use the challenge to uncover research opportunities and spark the interest of computer vision and AI researchers working on more robust and broadly usable visual reasoning models in the future. An interdisciplinary panel of speakers will further provide an
opportunity for fostering a mutual discussion between accessibility, computer vision, and robotics researchers and practitioners.
[ East Ballroom B ]
Creative domains render a big part of modern society, having a strong influence on the economy and cultural life. Much effort within creative domains, such as fashion, art and design, center around the creation, consumption, manipulation and analytics of visual content. In recent years, there has been an explosion of research in applying machine learning and computer vision algorithms to various aspects of the creative domains. For four years in a row, CVFAD workshop series have been capturing important trends and new ideas in this area. At CVPR 2023, we will continue to bring together artists, designers, and computer vision researchers and engineers. We will keep growing the workshop itself to be a space for conversations and idea exchanges at the intersection of computer vision and creative applications.
[ East 10 ]
Extracting health-related metrics is an emerging computer vision research topic that has grown rapidly recently. Without needing physical contact, cameras have been used to measure vital signs remotely (e.g. heart & respiration rates, blood oxygenation saturation, body temperature, etc.) from images/video of the skin or body. This leads to contactless, continuous and comfortable heath monitoring. Cameras can also leverage computer vision and machine learning techniques to measure human behaviors/activities and high-level visual semantic/contextual information, facilitating better understanding of people and scenes for health monitoring and provides a unique advantage compared to the contact bio-sensors. RF (Radar, WiFi, RFID) and acoustic based methods for health monitoring have also been proposed. The rapid development of computer vision and RF sensing also give rise to new multi-modal learning techniques that expand the sensing capability by combining two modalities, while minimizing the need of human labels. The hybrid approach may further improve the performance of monitoring, such as using the camera images as beacon to gear human activity learning for the RF signals. Contactless monitoring will bring a rich set of compelling healthcare applications that directly improve upon contact-based monitoring solutions and improve people’s care experience and quality of life, such as in care …
[ West 208 - 209 ]
Large Transformer models have performed promisingly on a wide spectrum of AI and CV applications. These positive performances have thus stimulated a recent surge of extremely large models. However, training these models generally requires more computation and training time. This has generated interest in both academia and industry in scaling up deep learning (DL) using distributed training on high-performance computing (HPC) resources like TPU and GPU clusters.
However, continuously adding more devices will not scale training as intended, since training at a large scale requires overcoming both algorithmic and systems-related challenges. This limitation prevents DL and CV researchers from exploring more advanced model architectures.
Many existing works investigate and develop optimization techniques that overcome these problems and accelerate large model training at a larger-scale. We categorize these works as improving either model accuracy or model efficiency. One method to maintain or improve model accuracy in a large-scale setting, while still maintaining computing efficiency, is to design algorithms that require less communication and memory demands. It is notable that these are not mutually exclusive goals but can be optimized together to further accelerate training. This tutorial helps enable CV members to quickly master optimizations for large-scale DL training and successfully train …
[ West 215 - 216 ]
4D Light fields can capture both intensity and directions of light rays, and record 3D geometry in a convenient and efficient manner. In the past few years, various areas of research are trying to use light fields to obtain superior performance internal structure information. Light fields have been widely used with remarkable results in some applications like depth estimation, super-resolution and so on. While the attempts in other applications like object detection and semantic segmentation are still in preliminary stage due to the lack of corresponding datasets, and incompatibility between redundant context information and limited memory. Meanwhile, more and more novel and powerful technologies like Neural Radiance Fields and Multiplane Image have been introduced into computer vision, there will be plenty of opportunities and challenges to incorporate them with light fields. To this end, this workshop focuses on two brand new topics. The first is to introduce the light field into more application areas, break through the bottleneck between rich structural information and limited memory, and achieve stable performance. The second is to explore how to introduce emerging technologies from other research fields into light fields to create new technological effects and drive competition. Besides, this workshop also hosts competitions …
[ West 301 ]
Pixel-level Scene Understanding is one of the fundamental problems in computer vision, which aims at recognizing object classes, masks and semantics of each pixel in the given image. Since the real-world is actually video-based rather than a static state, learning to perform video semantic/panoptic segmentation is more reasonable and practical for realistic applications. To advance the semantic/panoptic segmentation task from images to videos, we present two large-scale datasets (VSPW and VIPSeg) and a competition in this workshop, aiming at performing the challenging yet practical Pixel-level Video Understanding in the Wild (PVUW).
[ West 209 ]
This workshop is dedicated to event-based cameras, smart cameras, and algorithms processing data from these sensors. Event-based cameras are bio-inspired sensors with the key advantages of microsecond temporal resolution, low latency, very high dynamic range, and low power consumption. Because of these advantages, event-based cameras open frontiers that are unthinkable with standard frame-based cameras (which have been the main sensing technology for the past 60 years). These revolutionary sensors enable the design of a new class of algorithms to track a baseball in the moonlight, build a flying robot with the agility of a bee, and perform structure from motion in challenging lighting conditions and at remarkable speeds. These sensors became commercially available in 2008 and are slowly being adopted in computer vision and robotics. In recent years they have received attention from large companies, e.g., the event-sensor company Prophesee collaborated with Intel and Bosch on a high spatial resolution sensor, Samsung announced mass production of a sensor to be used on hand-held devices, and they have been used in various applications on neuromorphic chips such as IBM’s TrueNorth and Intel’s Loihi. The workshop also considers novel vision sensors, such as pixel processor arrays (PPAs), which perform massively parallel processing …
[ Virtual (AM); West 114 - 115 (PM) ]
Over the past years, mobile AI-based applications are becoming more and more ubiquitous. Various deep learning models can now be found on any mobile device, starting from smartphones running portrait segmentation, image enhancement, face recognition and natural language processing models, to smart-TV boards coming with sophisticated image super-resolution algorithms. The performance of mobile NPUs and DSPs is also increasing dramatically, making it possible to run complex deep learning models and to achieve fast runtime in the majority of tasks.
While many research works targeted at efficient deep learning models have been proposed recently, the evaluation of the obtained solutions is usually happening on desktop CPUs and GPUs, making it nearly impossible to estimate the actual inference time and memory consumption on real mobile hardware. To address this problem, we introduce the first Mobile AI Workshop, where all deep learning solutions are developed for and evaluated on mobile devices.
Due to the performance of the last-generation mobile AI hardware, the topics considered in this workshop will go beyond the simple classification tasks, and will include such challenging problems as image denoising, HDR photography, accurate depth estimation, learned image ISP pipeline, real-time image and video super-resolution. All information about the challenges, papers, …
[ West 306 ]
The Workshop has a unique aspect of fostering cross-pollination of different disciplines, bringing together experts (from academia & industry) and researchers of computer vision and pattern recognition, AI, machine learning, HCI, multimedia, robotics and psychology. The diversity of human behavior, the richness of multi-modal data that arises from its analysis, and the multitude of applications that demand rapid progress in this area ensure that our event provides a timely and relevant discussion and dissemination platform.
The workshop includes keynote talks from Prof. Gunes and Prof. Lapedriza, as well as presentations from experts and researchers within academia and industry on topics related to affective computing and behavior analysis.
The detailed agenda of the workshop can be found on the workshop's website.
[ East 14 ]
[ West 212 ]
[ East 10 ]
High-throughput microscopy enables researchers to acquire thousands of images automatically over a matter of hours. This makes it possible to conduct large-scale, image-based experiments for biological discovery. The main challenge and bottleneck in such experiments is the conversion of “big visual data” into interpretable information and hence discoveries. Visual analysis of large-scale image data is a daunting task. Cells need to be located and their phenotype (e.g., shape) described. The behaviors of cell components, cells, or groups of cells need to be analyzed. The cell lineage needs to be traced. Not only do computers have more “stamina” than human annotators for such tasks, they also perform analysis that is more reproducible and less subjective. The post-acquisition component of high-throughput microscopy experiments calls for effective and efficient computer vision techniques.
This workshop will bring together computer vision experts from academia, industry, and government who have made progress in developing computer vision tools for microscopy image analysis. It will provide a comprehensive forum on this topic and foster in-depth discussion of technical and application issues as well as cross-disciplinary collaboration. It will also serve as an introduction to researchers and students curious about this important and fertile field.
[ East 2 ]
The tutorial covers the task of visual localization, i.e., the problem of estimating the position and orientation from which a given image was taken. The tutorial’s scope includes cases with different spatial/geographical extent, small indoor/outdoor scenes, city-level, and world-level, and localization under changing conditions. In the coarse localization regime, the task is typically handled via retrieval approaches, which is covered in the first part of the tutorial. A typical use case is the following: Given a database of geo-tagged images, the goal is to determine the place depicted in a new query image. Traditionally, this problem is solved by transferring the geo-tag of the most similar database image to the query. The major focus of this part is on the visual representation models used for retrieval, where we include both classical feature-based and recent deep learning-based approaches. The 2nd and 3rd part of the tutorial encompass methods for precise localization with features-based and deep learning approaches, respectively. A typical use-case for these algorithms is to estimate the full 6 Degree-of-Freedom (6DOF) pose of a query image, i.e., the position and orientation from which the image was taken, for applications such as robotics, autonomous vehicles (self-driving cars), Augmented / Mixed / …
[ East 11 ]
Object localization in images is a key problem in a wide range of application domains that are embedded in critical settings such as self-driving vehicles or healthcare. However, most efficient solutions able to perform an object localization task follow the standard object detection and semantic segmentation frameworks, meaning that they require large amounts of annotated data for training. Different heuristics and tools can now assist and enhance human annotators, however manual annotation remains a largely heavy and expensive process. Moreover, perception models based on annotations enter a dependence circle of additional annotations for every new object class to detect or new external conditions to cover, e.g. in/outdoor, different times of the day, weathers. Such models struggle in dealing with our open complex world that is evolving continuously. Recent works have shown exciting prospects of avoiding annotations altogether by (1) leveraging self-supervised features, (2) building self-supervised object-centric objectives and (3) combining different modalities. In this context, we propose a half-day tutorial in which we will provide an in-depth coverage of different angles on performing/building-upon object localization with no human supervision.
[ East 16 ]
Visual understanding at different levels of granularity has been a longstanding problem in the computer vision community. The tasks span from image-level tasks (e.g., image classification, image-text retrieval, image captioning, and visual question answering), region-level localization tasks (e.g., object detection and phrase grounding), to pixel-level grouping tasks (e.g., image instance/semantic/panoptic segmentation). Until recently, most of these tasks have been separately tackled with specialized model designs, preventing the synergy of tasks across different granularities from being exploited.
In light of the versatility of transformers and inspired by large-scale vision-language pre-training, the computer vision community is now witnessing a growing interest in building general-purpose vision systems, also called vision foundation models, that can learn from and be applied to various downstream tasks, ranging from image-level , region-level, to pixel-level vision tasks.
In this tutorial, we will cover the most recent approaches and principles at the frontier of learning and applying vision foundation models, including (1) learning vision foundation models from natural language supervision, with applications to open-vocabulary image classification and retrieval, object detection, segmentation, and multimodal understanding; (2) learning vision foundation models via masked image modeling, with its extensions to multimodal pre-training; and (3) vision foundation model architecture design with transformer and …
[ West 205 - 206 ]
The half-day Women in Computer Vision (WiCV) workshop is a gathering for researchers of all genders and career stages. All are welcome and encouraged to attend the workshop. Topics include - wide range of areas, including object recognition, image understanding, video analysis, 3D reconstruction, etc.
Virtual Poster Session from 12:15 - 1:00 pm at https://topia.io/wicvcvpr2023
[ West 213 ]
Embedded vision is an active field of research, bringing together efficient learning models with fast computer vision and pattern recognition algorithms. We tackle many areas of robotics and intelligent systems and enjoy an impressive growth today.
[ West 217 - 219 ]
Federated Learning (FL) has become an important privacy-preserving paradigm in various machine learning tasks. However, the potential of FL in computer vision applications, such as face recognition, person re-identification, and action recognition, is far from being fully exploited. Moreover, FL has rarely been demonstrated effectively in advanced computer vision tasks such as object detection and image segmentation, compared to the traditional centralized training paradigm. This workshop aims at bringing together researchers and practitioners with common interests in FL for computer vision and studying the different synergistic relations in this interdisciplinary area. The day-long event will facilitate interaction among students, scholars, and industry professionals from around the world to discuss future research challenges and opportunities.
[ West 107 - 108 ]
The rapid development of computer vision algorithms increasingly allows automatic visual recognition to be incorporated into a suite of emerging applications. Some of these applications have less-than-ideal circumstances such as low-visibility environments, causing image captures to have degradations. In other more extreme applications, such as imagers for flexible wearables, smart clothing sensors, ultra-thin headset cameras, implantable in vivo imaging, and others, standard camera systems cannot even be deployed, requiring new types of imaging devices. Computational photography addresses the concerns above by designing new computational techniques and incorporating them into the image capture and formation pipeline. This raises a set of new questions. For example, what is the current state-of-the-art for image restoration for images captured in non-ideal circumstances? How can inference be performed on novel kinds of computational photography devices?
Continuing the success of the 1st (CVPR'18), 2nd (CVPR'19), 3rd (CVPR'20), 4th (CVPR'21), and 5th (CVPR'22) UG2 Prize Challenge workshops, we provide its 6th version for CVPR 2023. It will inherit the successful benchmark dataset, platform and evaluation tools used by the previous UG2 workshops, but will also look at brand new aspects of the overall problem, significantly augmenting its existing scope.
[ West 111 - 112 ]
This joint full-day workshop is the longstanding event that brings together the strongly growing egocentric computer vision community, offering the 3rd Ego4D edition and the 11th Egocentric Perception, Interaction and Perception (EPIC) edition. This year, 17 Ego4D benchmark and 9 EPIC benchmark winners and findings will be presented throughout the day, ranging from social interactions, episodic memory, hand-object interactions, long-term tracking, video object segmentations and audio-based interaction recognition. In addition to the recurring Ego4D and EPIC challenges, new challenges are associated with recently released benchmarks EgoTracks, PACO, EPIC-KTICHENS VISOR and EPIC-Sounds.
Additionally, the day will include accepted abstracts, invited CVPR papers and 5 keynotes by Andrea Vedaldi (Oxford and Meta), Hyun Soo Park (UMinnesota), David Fouhey (UMich) and Suraj Nair (Stanford). Check the program for details.
[ West 208 ]
The VISION workshop aims to provide a platform for the exchange of scholarly innovations and emerging practical challenges in Vision-based Industrial Inspection. Through a series of keynote talks, technical presentations, and challenge competition, this workshop is intended to (i) bring together researchers from the interdisciplinary research communities related to computer vision-based inspection; (ii) connect researchers and industry practitioners to synergize recent research progress and current needs in industrial practice.
[ East 19 - 20 ]
Polarization is a fundamental property of light and describes the direction in which the electric field of light oscillates. Polarization, as an intrinsic property of light, provides an extra dimension of information for probing the physical world. Although polarization is often overlooked, it allows for efficient geometry and material analysis beyond the conventional color images. With the snapshot quad-Bayer polarization camera being commercialized, there have been growing interests in using polarization cues to solve a wide range of computer vision problems. Recent advances have demonstrated advantages of using polarization imaging for geometry and material understanding.
In this tutorial, we will cover comprehensive topics in polarization imaging, from the fundamental physical principles to its applications in various computer vision problems. We will specifically focus on recent advances on using polarization imaging for solving the problems of reflectance modeling, 3D reconstruction, and transparent object segmentation. Finally, we will showcase applications of polarization imaging in industry settings.
[ East 8 ]
Does knowledge still have value in current era of large-scale pretraining? In this tutorial, we will comprehensively review existing paradigms for multimedia knowledge discovery and encoding, and focus on their contributions to vision-language pretraining. We categorize the knowledge into internal self-knowledge and external knowledge. Internal knowledge are extracted from text and vision modalities, such as structured entities, relations, events, and event procedures. We will focus on the structural aspects of the knowledge and address two key challenges regarding the acquisition of knowledge and encoding of structure across multiple modalities. External knowledge can be obtained from knowledge bases or language models, and we will exemplify their use to assist in commonsense understanding of vision modalities, with a focus on the temporal and cognitive aspects. The objective of this tutorial is to introduce participants to recent trends and emerging challenges in knowledge-driven vision-language research, as well as learning resources and tools for participants to obtain ready-to-use models, prompting thorough discussions regarding the impact of structured knowledge on text and vision learning.
[ East 5 ]
There is a growing trend of research in few-shot learning (FSL), which involves adapting learned knowledge to learn new concepts with limited few-shot training examples. This tutorial comprises several talks, including an overview of few-shot learning by Dr. Da Li and a discussion of seminal and state-of-the-art meta-learning methods for FSL by Prof. Timothy Hospedales. The tutorial will cover both gradient-based and amortised meta-learners, as well as some theory for meta-learning, and Dr. Yanwei Fu will introduce recent FSL techniques that use statistical methods, such as exploiting the support of unlabeled instances for few-shot visual recognition and causal inference for few-shot learning. Dr. Yu-Xiong Wang will also discuss various applications of FSL in fields beyond computer vision, such as natural language processing, reinforcement learning, and robotics.
[ West 116 - 117 ]
Learning in computer vision is all about deep networks and such networks operate on Euclidean manifolds by default. While Euclidean space is an intuitive and practical choice, foundational work on non-visual data has shown that when information is hierarchical in nature, hyperbolic space is superior, as it allows for an embedding without distortion. A core reason is because Euclidean distances scale linearly as a function of their norm, while hyperbolic distances grow exponentially, just like hierarchies grow exponentially with depth. This initial finding has resulted in rapid developments in hyperbolic geometry for deep learning.
Hyperbolic deep learning is booming in computer vision, with new theoretical and empirical advances with every new conference. But what is hyperbolic geometry exactly? What is its potential for computer vision? And how can we perform hyperbolic deep learning in practice? This tutorial will cover all such questions. We will dive into the geometry itself, how to design networks in hyperbolic space, and we show how current literature profits from learning in this space. The aim is to provide technical depth while addressing a broad audience of computer vision researchers and enthusiasts.
[ West 114 - 115 ]
This half-day tutorial will cover the latest advances in the broad theme of Optics for Better AI, with a specific focus on how to capture and synthesize realistic data for training low-light enhancement deep models. In this tutorial, we will first present the overall pipeline and effects of using realistic data, including (i) Low-light Image Enhancement using Synthesized Data; (ii) Low-light Video Enhancement using Captured Data. Then, we show detailed instructions on noise calibration and construction of optical imaging systems, including (iii) How to Calibrate the Noise Model of a Specific Camera; (iv) How to Construct a Co-axial Imaging System.
[ West 113 ]
Real-world applications of deep learning often have to contend with objectives beyond predictive performance, i.e., more than one equally important and competing objective or criterion. Examples include cost functions pertaining to invariance (e.g., to photometric or geometric variations), semantic independence (e.g., to age or race for face recognition systems), privacy (e.g., mitigating leakage of sensitive information), algorithmic fairness (e.g., demographic parity), generalization across multiple domains, computational complexity (FLOPs, compactness), to name a few. In such applications, achieving a single solution that simultaneously optimizes all objectives is no longer feasible; instead, finding a set of solutions that are representative in describing the trade-off among objectives becomes the goal. Multiple approaches have been developed for such problems, including simple scalarization and population-based methods. This tutorial aims to provide a comprehensive introduction to fundamentals, recent advances, and applications of multi-objective optimization (MOO), followed by hands-on coding examples. Some emerging applications of MOO include (1) hardware-aware neural architecture search; (2) multi-task learning as multi-objective optimization; (3) representation learning for privacy and fairness. We will also summarize potential research directions intersecting MOO and ML/CV research.
[ West 302 - 305 ]
A full day tutorial covering all aspects of autonomous driving. This tutorial will provide the necessary background for understanding the different tasks and associated challenges, the different sensors and data sources one can use and how to exploit them, as well as how to formulate the relevant algorithmic problems such that efficient learning and inference is possible. We will first introduce the self-driving problem setting and a broad range of existing solutions, both top-down from a high-level perspective, as well as bottom-up from technological and algorithmic points of view. We will then extrapolate from the state of the art and discuss where the challenges and open problems are, and where we need to head towards to provide a scalable, safe and affordable self-driving solution for the future.
[ East 7 ]
This tutorial will deliver a well-rounded understanding of the emerging field of reverse engineering of deception (RED) techniques, a cutting-edge topic in adversarial machine learning (ML) for reliable computer vision (CV). Past studies have extensively explored the generation, detection, and defense of machine-centric deception (e.g., adversarial attacks that deceive ML models) and human-centric deception (e.g., GAN-created images that mislead human decision-making) in CV. However, RED introduces a new adversarial learning paradigm that automatically uncovers and catalogs attack "fingerprints" found in both machine and human-centric attacks. The RED problem addressed in the tutorial is: Can we reverse-engineer the adversary's knowledge and attack toolchains beyond conventional adversarial detection/defense techniques? To this end, this tutorial will cover the following key aspects: (1) Review RED's definition and formulation, addressing basics and preliminaries. (2) Discuss the challenges and significance of RED, highlighting its connections and differences with conventional adversarial detection/defense techniques in ML. (3) Explore RED for machine-centric adversaries, reviewing recent RED developments on top of a variety of adversarial attacks. (4) Examine RED for human-centric adversaries, reviewing RED methods for the detection and model parsing of GAN-generated fake images. (5) Demonstrate and showcase RED applications in CV.
[ East 17 ]
This half-day tutorial will cover the latest advances in this area from three aspects, i.e., motion modeling and optimization-based solutions, deep learning-based solutions, and joint hardware and deep learning-based solutions. Specifically, we will first systematically present geometric motion models (like discrete, continuous, and special motions) and optimization-based approaches. Then, we will introduce deep learning-based RS image processing methods, such as RS image correction and RS temporal super-resolution, with new results and benchmarks that have recently appeared. Finally, we will elaborate on the combination of hardware features of RS cameras (e.g., dual RS cameras and global reset feature) and deep learning to boost the correction of RS geometric distortions.
[ East 15 ]
Creating high-level structured 3D models of real-world indoor scenes from captured data and exploiting them are fundamental tasks with important applications in many fields. In this context, 360 capture and processing is very appealing, since panoramic imaging provides the quickest and most complete per-image coverage and is supported by a wide variety of professional and consumer capture devices. Research on inferring 3D indoor models from 360 images has been thriving in recent years, and has led to a variety of very effective solutions. Given the complexity and variability of interior environments, and the need to cope with noisy and incomplete captured data, many open research problems still remain. In this tutorial, we provide an up-to-date integrative view of the field. After introducing a characterization of input sources, we define the structure of output models, the priors exploited to bridge the gap between imperfect input and desired output, and the main characteristics of geometry reasoning and data-driven approaches. We then identify and discuss the main subproblems in structured reconstruction, and review and analyze state-of-the-art solutions for floor plan segmentation, bounding surfaces reconstruction, object detection and reconstruction, integrated model computation, and visual representation generation. We finally point out relevant research issues and …
[ West 211 ]
What is the interplay of width/depth and how does the initialization affects the robustness to adversarial attacks? What is a principled heuristic for selecting good architectures in Neural Architecture Search (NAS)? What is the role of Fourier features in implicit neural representations (INRs)? In this tutorial, we aim to build a bridge between the empirical performance of neural networks and deep learning theory. In particular, we want to make the recent deep learning (DL) theory developments accessible to vision researchers, and motivate vision researchers to design new architectures and algorithms for practical tasks. In the first part of the tutorial, we will discuss popular notions in DL theory, such as lazy training and Neural Tangent Kernel (NTK), or bilevel optimization for adversarial attacks and NAS. Then, we will exhibit how such tools can be critical in understanding the inductive bias of networks.
[ West 223 - 224 ]
Originating from natural language processing, the new paradigm of prompting has recently swept through the computer vision community, bringing disruptive changes to various computer vision applications, such as image recognition and image generation. In comparison to the traditional fixed-once-learned architecture, like a linear classifier trained to recognize a specific set of categories, prompting offers greater flexibility and more opportunities for novel applications. It allows the model to perform new tasks, such as recognizing new categories, by tuning textual instructions or modifying a small number of parameters in the model's input space while keeping the majority of the pre-trained parameters untouched. This paradigm significantly pushes conversational human-AI interaction to unprecedented levels. Within a short period of time, the effectiveness of prompting has been demonstrated in a wide range of problem domains, including image classification, object detection, image generation and editing, video analytics, and robot control. In this tutorial, our aim is to provide a comprehensive background on prompting by building connections between research in computer vision and natural language processing. We will also review the latest advances in using prompting to tackle computer vision problems.
[ East Ballroom B ]
State-of-the-art computer vision systems are trained to predict a fixed set of predetermined object categories. This restricted form of supervision limits their generality and usability since additional labeled data is needed to specify any other visual concepts.
Recent works show that learning from large-scale image-text data is a promising approach to building transferable visual models that can effortlessly adapt to a wide range of downstream computer vision (CV) and multimodal (MM) tasks. For example, CLIP, ALIGN and Florence for image classification, ViLD, RegionCLIP, GLIP and OWL-ViT for object detection, GroupViT, OpenSeg, MaskCLIP, X-Decoder, Segment Anything (SAM) and SEEM for segmentation, Multimodal GPT-4, LLaVA and MiniGPT4 for langauge-and-image instruction-following chat assistants. These vision models with language or interactive interface are naturally open-vocabulary recogntion models, showing superior zero-shot and few-shot adaption performance on various real-world scenarios.
We host this "Computer Vision in the Wild (CVinW)" workshop, aiming to gather academic and industry communities to work on CV and MM problems in real-world scenarios, focusing on the challenge of open-set/domain visual recognition at different granularities and efficient task-level transfer. To measure the progress of CVinW, we develop new benchmarks for image classification, object detection and segmentation to measure the task-level transfer ablity of …
[ East 12 ]
The Visual Copy Detection Workshop (VCDW) explores the task of identifying copied images and videos, robust to common transformations. This task is central to social problems facing online services where users share media, such as combating misinformation and exploitative imagery, as well as enforcing copyright. Recently, copy detection methods have been used to identify and promote original content, and to reduce memorization in both predictive and generative models.
The workshop will explore technical advances in copy detection as well as the applications that motivate this research. The workshop will feature the Video Similarity Challenge, a copy detection challenge in the video domain, including presentations by challenge participants.
[ East 3 ]
[ East 2 ]
Our objective is to provide a venue for novel research in omnidirectional computer vision with an eye toward actualizing these ideas for commercial or societal benefit. As omnidirectional cameras become more widespread, we want to bridge the gap between the research and application of omnidirectional vision technologies. Omnidirectional cameras are already widespread in a number of application areas such as automotive, surveillance, photography, simulation and other use-cases that benefit from large field of view. More recently, they have garnered interest for use in virtual and augmented reality. We want to encourage the development of new models that natively operate on omnidirectional imagery as well as close the performance gap between perspective-image and omnidirectional algorithms.
[ West 116 - 117 ]
[ West 109 - 110 ]
[ East 12 ]
Project Aria is a research device from Meta, which is worn like a regular pair of glasses, and enables researchers to study the future of always-on egocentric perception. In this tutorial, we will introduce two exciting new datasets from Project Aria: Aria Digital Twin, a real-world dataset with hyper-accurate digital counterpart; and Aria Synthetic Environments, a procedurally-generated synthetic Aria dataset for large-scale ML research. Each dataset will be presented with corresponding challenges, which we believe will be powerful catalysts for research. In addition to introducing new datasets and research challenges, we will also provide a hands-on demonstration of newly open-sourced tools for working with Project Aria, and demonstrate how the Project Aria ecosystem can be used to accelerate open research into egocentric perception tasks such as visual and non-visual localization and mapping, static and dynamic object detection and spatialization, human pose and eye-gaze estimation, and building geometry estimation.
[ East 11 ]
This tutorial focuses on describing techniques to allow deep learning practitioners to accelerate the training and inference of large deep networks while also reducing memory requirements across a spectrum of off-the-shelf hardware for important applications such as autonomous driving and large language models. Topics include, but are not limited to: 1) Deep learning specialized hardware overview. We review the architecture of the most used deep learning acceleration hardware, including the main computational processors and memory modules. 2) How deep learning is performed on this hardware. We cover aspects of algorithmic intensity and an overview of theoretical aspects of computing. Attendees will learn how to estimate processing time and latency by looking only at hardware specs and the network architecture. 3) Best practices for acceleration. We provide an overview of best practices for designing efficient neural networks including channel number selection, compute heavy operations, or reduction operations among others. 4) Existing tools for model acceleration. In this part we will focus on existing tools to accelerate a trained neural network on GPU devices. We will particularly discuss operation folding, TensorRT, ONNX graph optimization, sparsity. 5) Research overview of recent techniques. In the last part, we will focus on recent advanced techniques …
[ East 18 ]
With the rise of edge computing, increase in remote sensing information, and ubiquitous adoption of computer vision systems throughout retail and manufacturing markets, organizations are increasingly relying on the accuracy and reliably of training Artificial Intelligence and Machine Learning systems to analyze and extract information from data captured using physical sensors and sensor platforms. Real data sets often fail to capture rare events or assets, are inaccurately labeled, and the collection of real sensor data can have cost, privacy, security, and safety issues.
Synthetic data offers the opportunity to design and label datasets for specific algorithmic training needs. Synthetic imagery designed to emulate ground-based video systems or remotely sensed satellite imagery, for example, can be generated to show real world locations populated with objects that are hard to find or that don’t yet exist. Accurately labeled, simulated datasets can be created to fit a wide range of potential real-world scenarios in which AI/ML systems will be deployed, thereby enabling teams to train and test these systems before being deployed in production environments.
This tutorial will include an introduction to creating, using, and iterating on synthetic data using the open Rendered.ai synthetic data platform. We will also feature a demonstration using …
[ West 113 ]
Neural search, a technique for efficiently searching for similar items in deep embedding space, is the most fundamental technique for handling large multimodal collections. With the advent of powerful technologies such as foundation models and prompt engineering, efficient neural search is becoming increasingly important. For example, multimodal encoders such as CLIP allow us to convert various problems into simple embedding-and-search. Another example is the way to feed information into LLMs; currently, vector search engines are a promising direction. Despite the above attention, it is not obvious how to design a search algorithm for given data. In this tutorial, we will focus on "million-scale search", "billion-scale search", and "query language" to show how to tackle real-world search problems.
[ East 8 ]
[ West 118 - 120 ]
Join our social event to get the tools, information, and data you need to negotiate your next offer more confidently. Some of the topics we'll cover in a 2 hour period (including 45 mins for Q&A) are: Understanding the fundamentals of compensation in tech (particularly around equity, - bonus structures, etc.), data points for different levels/positions in AI, how to get over your fears of negotiating, how to decide which company / offer is right for you, how to negotiate without counter offers and without knowing "market value", how to respond to pushback from recruiters and other guilt tripping / lowballing / pressure tactics, how to avoid having an offer rescinded, how to negotiate deadline of an offer and walking through a timeline of the negotiation process for a new offer.
[ West 201 ]
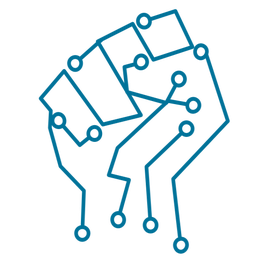
Africa has the second-largest population in the world with around 1.4 billion people as of 2022. With the increasing amount of visual data and the growing rate of its data footprint, the impact of extending Computer Vision research to solving local problems specific to Africa has become an ever-increasing need. This social event aims to bring together a unique community of people who self-identify as Black and/or from African origin or support the Black community at its first gathering in CVPR. Our main goal is to create a platform where Black researchers are comfortable meeting with other people without feeling out-of-place and to enforce a strong connection of like-minded individuals whose main or sub-goals is to empower the African community and Black Computer Vision researchers. This social, therefore, has several aims:
- Empowering Black and African origin researchers by affirming their sense of belonging to the Computer Vision community specifically in CVPR.
- Providing mentorship and guidance to young researchers from the Black and African origin community.
- Allowing both Black and African-origin researchers and their supporters/allies to gather and network within the Computer Vision community.
- Celebrating African grassroots in AI, especially in the field of Computer Vision.